Abstract
Agricultural supply chains play a crucial role in supporting food security in Africa. However, high-resolution supply chain information is often not available, which hinders our ability to determine which interventions in food supply chains would most enhance food security. In this study, we develop a high-resolution supply chain model for essential staple crops in Zambia, aiming to estimate how improvements in transportation infrastructure would impact food security. Specifically, we simulate district-level monthly consumption, trade flows, and storage for maize and cassava in Zambia. We then conduct a counterfactual case study with low transportation costs, discovering that reducing transaction costs leads to higher aggregate net agricultural revenue and aggregate net expenditure. These results indicate that transportation investments are more beneficial to suppliers than to consumers, with implications for household food security in smallholder agriculture. Our study highlights the potential for infrastructure investments to improve food security.
Export citation and abstract BibTeX RIS

Original content from this work may be used under the terms of the Creative Commons Attribution 4.0 license. Any further distribution of this work must maintain attribution to the author(s) and the title of the work, journal citation and DOI.
1. Introduction
Sub-Saharan Africa remains the world's most food insecure region (WFP, WHO and UNICEF 2022). Throughout much of Sub-Saharan Africa, agriculture is predominantly produced by smallholder farmers who rely on seasonal rainfall for crop cultivation, without irrigation to buffer shortfalls in the rains (Samberg et al 2016, Ricciardi et al 2018). Smallholder agriculture is particularly at risk from various climate and socioeconomic shocks, including droughts and floods, pests, political instability, and economic downturns, amongst others (Shiferaw et al 2009, Niles and Salerno 2018). Insufficient infrastructure, characterized by inadequate transport networks and limited grain storage facilities, further exacerbates the challenges faced by smallholders in Africa (Affognon et al 2015). While there has been extensive research on increasing crop productivity across Sub-Saharan Africa (Foley et al 2011, Mueller et al 2012, Leitner et al 2020), there has been comparatively less research focused on the role of food trade in mitigating food insecurity, particularly the impact of infrastructure.
Food trade is an important strategy for mitigating food insecurity in the face of growing food demand and supply volatility (Panagariya et al 2002, Baldos and Hertel 2015). Enhancing supply chains has the potential to foster greater food security against weather, economic, and political shocks (Chichaibelu et al 2021). Nonetheless, there is a significant knowledge gap regarding food trade, especially within developing countries, where spatialized data on sub-national trade flows is often incomplete or unavailable (Konar et al 2018). Most prior research on food trade and exchange has focused on either national-level or household-level analyses. National-level studies often examine trade patterns and policies between countries or regions, including the impact of trade agreements and tariffs on food security (Gayi et al 2007, Chikhuri 2013). In contrast, household-level studies often explore the dynamics of food exchange and sharing between households or communities, delving into the influence of social networks (Von Gnechten et al 2020). While these studies offer valuable insights into food trade and exchange across spatial scales, further research is needed to investigate food trade at the regional scale within countries. The regional scale trade information would enable planners to identify the specific infrastructure and logistical challenges facing domestic food supply chains. Therefore, we focus on district-level trade in Zambia.
Our contribution to the existing literature lies in the development of a comprehensive agricultural supply chain model for Zambia. We estimate the trade costs throughout the country based on spatialized price differences, following the approach proposed by Donaldson (2018). This enables us to establish the connection between trade costs and the transportation infrastructure, thereby identifying potential opportunities for future transportation planning. Importantly, our study incorporates a counterfactual analysis that explores the impact of reducing trade costs between districts throughout Zambia.
We focus on Zambia, a landlocked country in Southern-Central Africa whose agricultural system is dominated by rainfed smallholders. Despite experiencing food surpluses in most years, Zambia continues to face persistent food shortages and widespread hunger in various regions (Chapoto et al 2019). The country's food security faces significant challenges due to increasing urbanization, food price inflation, and the increasing frequency of extreme weather (Simatele and Simatele 2015, Mulenga 2016). Previous trade models of Zambia have mainly concentrated on the large (national) scale and small (household) scale, whereas this study simulates the meso-scale (districts). Von Gnechten et al (2020) examined household food sharing in Zambia and observed seasonal fluctuations in sharing. Porteous (2019) modeled agricultural storage and trade across Sub-Saharan Africa, revealing that high trade costs significantly hinder the potential for trade to enhance food security. Dorosh et al (2007) utilized a demand and supply economic model to estimate the flow of food between southern and northern Zambia and suggested that private cross-border trade and the substitution of cassava could help moderate food volatility.
Our model is designed to capture the characteristics of the Zambian food system. We focus on maize and cassava, the primary calorie sources for the Zambian population, contributing 46% and 25% respectively, as per the FAO 2020 food balance sheet (FAO 2023). Furthermore, the model distinguishes between public and private maize supply chains in different seasons, both of which are prevalent in the Zambian food system (Mason et al 2015). The harvest season here refers to the maize harvest season. The lean season, which typically occurs between December and March in Zambia, represents a period when food stocks are typically low. Figure 1 abstracts the behavior of different stakeholders in the Zambia staple market. The private channel involves briefcase businessmen, large companies, and millers that engage in buying and/or selling crop commodities for example maize and cassava between regions. On the other hand, the public channel refers to the government's maize procurement program, led by the Food Reserve Agency (FRA) to manage strategic national maize reserves (Food Reserve Agency 2022). By characterizing both private and public supply chains in different seasons, our model provides a comprehensive understanding of the maize trade in Zambia. During harvest season, rural households are net producers and sell maize to different channels, eventually reaching urban households (consumers). However, during some lean seasons, some rural households become net consumers, needing maize support from big millers, who might obtain maize at a subsidized price from the FRA. This highlights that the relationship between producers and consumers varies over the agricultural calendar, which is a major reason that we run our model at the month time step. The supply chain model enables us to identify those interventions that have the potential to enhance food security and alleviate poverty.
Figure 1. Conceptual diagram of interactions between actors in the maize and cassava supply chains in Zambia. During the harvest season, farmers will sell the maize to the Food Reserve Agency as (1), and with the help of private briefcases, they can also sell the maize to big millers as (2), and urban areas where there is a lack of production of maize as (3) and (6). Some farmers loan money or have some contracts with big companies like CARGILL in lean season and the farmers need to send the maize to the companies according to the contract in harvest season as (4). The companies will sell the staples to big millers (4) and foreign markets (5). During the lean season, the Food Reserve Agency might get a signal of high price of maize as (7), and then they will release the maize public storage to nearby big millers as (8) and even import maize when they lack public storage as (9). The millers redistribute the maize to regions that suffer from hunger (generally with a higher maize spot price) as (6) and (10). The figure is created by the authors.
Download figure:
Standard image High-resolution imageThe objective of this study is to develop a supply chain model for maize and cassava, which will be evaluated by comparing the prices generated by the model with the surveyed monthly district-level prices. A wide range of primary and secondary input data from 2010 to 2020 is utilized to develop the model, as outlined in table 2. The model's output contributes to our understanding of public and private trading dynamics, consumer behavior, and storage decisions in Zambia, which lack detailed empirical evidence. We examine a hypothetical scenario where trade costs are reduced to answer our overarching research question: 'What is the role of infrastructure on food security in Zambia?' Specifically, we ask: 'What is the impact of reducing trade (or transportation) costs on food security in Zambia?' This could help decision-makers gain insights into the potential benefits of investing in transportation infrastructure. The supply chain model is elaborated in section 2. Section 3 presents the simulated flows, consumption, and storage for each district, as well as the counterfactual analysis for transportation cost reduction. Further applications and limitations of the model are shown in section 4. We conclude in section 5.
2. Dynamic regional agricultural supply chain model
2.1. Model theory
We build a partial equilibrium model to analyze the supply chains of maize and cassava. The monthly equilibrium of storage, trade, and consumption are solved when market clearing conditions (equation (1)), inverse demand (equation (8)), spatial arbitrage (equation (3)), and temporal arbitrage (equation (4)) are satisfied simultaneously. Table 1 lists the parameters and variables in this study for reference.
Table 1. Definition of variables in the Zambian agricultural supply chain model.
Symbol | Description | Role |
---|---|---|
![]() | 72 district-scale markets within Zambia; m for outflow of districts; | |
n for inflow of districts | ||
t | Time, in month | |
c | Crop, referring to maize and cassava | |
ε | Elasticity of demand for staple composite | CP |
Dm | Demand coefficient for each market m | CP |
αcm | Share parameter of crop c in market m | CP |
σ | Elasticity of substitution between maize and cassava | CP |
km | Per unit grain storage fee by market m | TP |
rm | Real interest rate of market m | TP |
κc | Trade cost multiplier for crop c | TP |
τmn | Relative per unit crop trade cost between market m and ROW | TP |
Nmt | Population for market m at time t | ExV |
Ymt | Total income of aggregate consumers in market m at time t | ExV |
Hcmt | Production of crop c in market m at time t | ExV |
Fmt | Public purchase/release of maize for market m at time t | ExV |
Qmt | Consumption of staple composite for market m catchment at time t | EnV |
Xmt | Consumption of the numeraire for market m catchment at time t | EnV |
Umt | Utility for market m catchment at time t | EnV |
Qcmt | Final consumption of crop c for market m catchment at time t | EnV |
Pcmt | Price of grain c for market m at time t | EnV |
Scmt | Private storage of grain c for market m at time t | EnV |
Tcmnt | Private trade volume of grain c from market m to market n at time t | EnV |
Consumers parameters (CP), traders parameters (TP), exogenous variables (ExV), endogenous variables (EnV).
2.1.1. Defining the system
Utilizing the theoretical framework described in section 2.1, we implement a supply chain model for Zambia spanning the years 2010 to 2020. The temporal resolution of the model is the monthly time period (t). The spatial resolution of the model is the district scale, representing the markets (m, n) within the model. We treat each individual district as a market catchment and study the behavior of agents in each district. Additionally, we incorporate international trade in our model between Zambian districts and a lumped 'Rest of World' entity. This means that 'model export' estimates exports from districts to the 'Rest of World'; 'model imports' are estimates of how much the 'Rest of World' sends to each district in Zambia.
The model focuses on two staple crops: maize and cassava (c). Each crop is treated as a homogeneous agricultural good in every market following the standard assumption in modeling staples within developing countries (Porteous 2019, Sotelo 2020). Other goods are collectively represented as an outside numeraire good.
In each market m, the behavior of four agents is vital: a representative producer, a representative consumer, a representative public trader, and a representative private trader. We assume the behavior of the representative producer is tractable, which means that the production of crops is treated as exogenous to the model. Incorporating external production into the model serves as a simplification of real-world dynamics. This simplification aligns with our objective to ascertain whether it is possible to enhance food security by lowering trade costs within the current production conditions. Within a market, the representative consumer and producer engage in transactions involving the buying and selling of crops at the district wholesale price. The representative public trader refers to the government crop procurement organization, the FRA. The representative private trader has the ability to purchase goods from market m and sell them in another market n, provided that there exists a transportation link between the two markets. The behavior of the representative consumer, public trade, and private trade is modeled by extending the fundamental rational expectations framework of Williams et al (1991) for each market.
2.1.2. Market clearing condition
The supply chain model must adhere to the market clearing condition, which ensures that the supply and demand in each market are in equilibrium. This condition is expressed by equation (1):

In equation (1), the left side represents the total supply available to the private trader in each market m. The supply consists of three components: local production (Hcmt
), FRA releases (Fcmt
), and staple carried over from the last period's storage (). On the right side of equation (1), private traders decide how to allocate this total supply. They decide the amount to be sold for local consumption (Qcmt
), the quantity to be stored for the next period (Scmt
), and the volume to be traded with other markets (Tcmnt
).
2.1.3. Representative private traders maximize profits
The private trader strategically allocates supply to maximize their profit each month, considering the storage cost, trade cost, current price 6 , time value of money, and rational expectations of future price. The mathematical expression for this profit maximization problem is shown in equation (2)

where represents the mathematical expectations operator, conditional on the information available at time t. km
represents the per-unit storage cost, rt
is the real interest rate at time t, κc
is the trade cost parameter for different crops, and τmn
denotes the relative trade cost between market m and n.
Replacing Qcmt with equation (1) and taking the first-order condition with respect to Tcmnt , we obtain the spatial arbitrage condition 7 , as shown in equation (3)

Taking the first-order condition with respect to Scmt yields the temporal arbitrage condition, as depicted in equation (4):

2.1.4. Representative consumers maximize utility
The representative consumers decide their monthly consumption of each crop and the numeraire good to maximize their utility given their budget constraint. We employ a two-stage budget procedure to estimate the demand for each good, assuming weak separability of consumer preferences for each crop compared to outside goods. In the first stage, consumers decide the allocation of their total expenditure between staples and outside goods. We assume a quasilinear utility function for the numeraire good, meaning that the first stage utility function is linear with respect to the outside goods and is strictly concave in the crop composite as illustrated by equation (5)

where, Umt represents consumer utility in market m at time t. Dm is a constant parameter for each market, and Nmt represents the population. Qmt refers to the consumption of the crop composite. Xmt represents the consumption of the numeraire. ε indicates the elasticity of demand for the staple composite.
In the second stage, the price of each crop and total expenditure on staples determine the consumption of each crop. The preferences for individual crops follow a constant elasticity of substitution (CES) model, as shown in equation (6)

where σ and αcm
are demand parameters indicating the elasticity of substitution and share parameters, respectively. The sum of the share parameters for crops in each market is normalized (i.e. ).
In summary, the behavior of representative consumers can be expressed by equation (7)

The representative consumers maximize their utility function subject to their income constraints. The inverse demand function for each crop in each market is obtained using the method of Lagrange multipliers, resulting in equation (8). The detailed derivation of equation (8) is provided in the supporting information (SI).

2.1.5. The public trader tries to stabilize maize prices
The FRA was assigned the responsibility of acquiring, storing, and subsequently distributing stocks in the market during periods of food scarcity and other types of disasters that lead to price fluctuations (Mukumba et al 2018). FRA follows a 'price-band' scheme to keep prices within a narrow range, which have not proven successful especially in the case of abundant or meager harvests. In each district, the FRA offers to purchase maize for the government stockpile 8 at a 'floor price', with the purchase target limited by financial constraints and local production. Generally, the FRA sells maize at subsidized prices during food stresses to large millers , which are often located in large cities, such as the capital city of Lusaka. The most vulnerable groups, for example the rural poor, could lose out from the differing purchase and release activities of FRA in urban and rural areas (Mason and Myers 2013). We decide to estimate the FRA as a separate entity in the model, rather than applying the general method for modeling the price band policy, due to these concerns. The annual district-level FRA purchase data is obtained from the FRA office and we take it as given (Food Reserve Agency 2021). We identify the locations of prominent millers and model the FRA maize flows to the major millers as a multi-source, multi-destination transportation problem using the linear programming, formulated as equation (9)
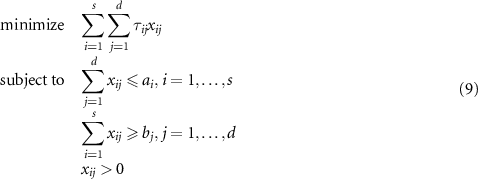
where the objective function aims to minimize the cost of trade between FRA stock i and millers j. The per unit transportation cost is denoted by τij
and the shipment amount is represented by xij
. Given the absence of rerouting, mass conservation has been accounted for by the two constraints: (1) the total outgoing shipment must not exceed the FRA stock in that market (ai
). The annual district-level FRA purchase data is provided by the FRA office. The initial stock for 2009 is assumed to be zero, and subsequent FRA stocks for each market (ai
) are calculated year-by-year. (2) The total incoming shipment must meet or exceed the demand of millers (bj
). The demand for each market is determined based on the daily capacity of local large milling plants. In our model, we focus solely on the aggregate flow and not individual truck deliveries, so we do not need to include an upper threshold. Subsequently, we estimate the monthly FRA purchase and release amounts , assuming that the purchases occur during the harvest month and the stocks are released evenly over the lean months.
2.2. Input data processing and analysis
We leverage several secondary datasets, government reports, and peer-reviewed papers to compile the required datasets for our model and conduct preliminary analyses. Table 2 provides all the datasets used in this study.
Table 2. A summary of datasets used in this study.
Name | Reference | Description |
---|---|---|
Zambia District Boundary | Hijmans (2015) | Since the division of districts in Zambia has changed since 2011 with the creation of new districts, we use the same district classification as it existed in 2010 throughout the study to ensure consistency in the results over time. The panel dataset is adjusted accordingly to align with the selected district boundaries. |
Monthly Zambia Commodity Price by District | Central Statistical Office of Zambia (2020) | This dataset provides monthly Zambia commodity price by district. Staple prices are used to validate the goodness of fit of this study in section 3.1. Sugar prices are used in section 2.3.2 to estimate the trade cost parameter. |
National Population | United Nations and Social Affairs (2022) | This dataset contains annual national population data from 2010 to 2020 |
Zambia Population Census (2010) | Central Statistical Office of Zambia (2019) | The Zambia population census in 2010 provides population data at the district level. The national population is allocated to each district, assuming that the district population ratio remains constant from 2010-2020, as detailed in section 2.2.2. |
Crop Forecast Survey (CFS) | Central Statistical Office of Zambia (2021) | Crop Forecast Survey provides annual district-level maize and cassava production estimates. It is referenced in section 2.2.3 to generate the production input. |
Crop Calendar | FAO (2021) | Crop Calendar shows the harvest time for four different agro-ecological zones in Zambia. We use the crop calendar in section 2.2.3 for maize to allocate harvest to different month for each district. |
Food Reserve Agency Data | Food Reserve Agency (2021) | FRA provides the annual maize procurement amount by district and national strategic reserve balance. Estimation of the public flows are solved as a multi-source multi-destination detailed in sections 2.1.5 and 2.2.4 and the flow amount serves as the input to the equilibrium model. |
Living Condition Monitoring Survey (LCMS) 2010 | Central Statistical Office of Zambia (2010) | LCMS includes the expenditure on maize and cassava which are used in section 2.3.1 to calculate the share parameters for each district. |
Open Street Map (OSM) | OpenStreetMap contributors (2022) | We construct the transportation network using the Open Street Map. The transportation network are used to calculate the trade cost parameters as detailed in section 2.3.2. |
2.2.1. Price dispersion across districts and over time
The monthly crop price series are collected over a span of 132 months, covering 72 markets from January 2010 to December 2020 (Central Statistical Office of Zambia 2020). The price series for maize and cassava exhibit incompleteness. Maize has only 7364 out of 9504 (77%) possible observations, while cassava has only 3293 out of 9504 (35%) possible observations, indicating a partial representation of the market dynamics for these crops. The original price series are wholesale prices in the local currency of Zambia (Kwacha, ZMK). To facilitate comparison and analysis, we convert the price series to USD/kg 9 using the monthly USD-ZMK exchange rate deflated by US Consumer Price Index to the base year 2010. Across all time periods and markets in Zambia, the average price of maize was 0.215 USD kg−1, and the average price for cassava flour/meal was 0.631 USD kg−1.
Figure 2 illustrates the substantial variation in crop price across districts and over time. This spatial and temporal variability in price is a key factor driving regional trade. The significant price dispersion indicates incomplete or costly arbitrage. Seasonal price variations are significant for both maize and cassava, therefore, a more detailed supply chain model at a finer time-scale could provide insights for storage management.
Figure 2. The average maize (A) and cassava (B) prices in each district. Monthly prices for selected districts between 2010 and 2020 for maize (5 districts; (C) and for cassava (3 districts; (D).
Download figure:
Standard image High-resolution imageInternational cassava trade is not considered in our model due to its minimal amount. The maize price for the Rest of World market is assumed to be the Johannesburg market price (SAFEX). The assumption is based on the fact that the majority of agricultural imports to Zambia originate from South Africa, which is a nearby maize-surplus country 10 . The average price for SAFEX maize is 0.202 USD kg−1.
2.2.2. Population growth across districts and through time
The annual national population is obtained from the UN Population Division (United Nations and Social Affairs 2022). To allocate the national-level population data to specific districts, we utilize the district-level population in 2010 from the Zambia population census (Central Statistical Office of Zambia 2019). We assume that the percentage of the national population residing in each district remained constant throughout the study period and that there are no changes in population within a given year. This approach enables us to derive monthly population data at the district level.
Population density varies spatially throughout Zambia, ranging from 3 people per km2 in Mufumbwe to 3681 people per km2 in Lusaka. The average population of Zambia during the study period is 15.9 million, representing a 35% increase from 2010 to 2020.
2.2.3. Crop productivity across districts and through time
The annual estimates of district-level maize production are obtained from the Crop Forecast Survey (CFS) (Central Statistical Office of Zambia 2021). Zambia is divided into four distinct agro-ecological zones (FAO 2021), each with varying climate conditions that result in different harvest times. Accordingly, we assign May as the harvest month of maize for districts in Region I, June for districts in Region IIa, January for districts in Region IIb, and April for districts in Region III. Consequently, production entries exist only for the assumed harvest month, while the remaining months have a value of zero.
The annual district-level cassava production data is derived from the CFS, with missing data recorded in the years 2006, 2019, and 2020. To address the missing cassava production, linear regression is utilized for interpolation. Given the versatile nature of cassava harvests, which can occur in any season depending on local grain reserves and prices, we assume a January harvest for cassava across all years. This assumption is made based on the prevalent practice of harvesting cassava during the lean season to mitigate food insecurity.
Figure 3 displays the distribution of per capita production across different regions of Zambia. The southern regions exhibit higher levels of per capita maize production compared to the northern regions. Notably, the district of Mpongwe records the highest production at 789 kg per capita, while Lusaka reports the lowest production at 0.81 kg per capita. According to Sitko et al (2011), Zambians consume an estimated 85 to 140 kg of maize per person annually. The variations in production levels among districts emphasize the significance of trade as a means to address the disparities.
Figure 3. Maize and cassava production in Zambia. Annual district-level total production (A) and mean productivity per capita of maize (B) and cassava (C).
Download figure:
Standard image High-resolution image2.2.4. Food Reserve Agency (FRA) maize procurement over time
Figure 4 presents the historical data depicting the government's maize procurement through the FRA over time. It is evident that FRA purchases have decreased over the years, resulting in an expanded role for private traders in the market.
Figure 4. Time series of Food Reserve Agency purchase volumes, procurement prices, and wholesale prices. Data source: Food Reserve Agency (2021).
Download figure:
Standard image High-resolution imageWe estimate the amount of maize released in areas with large millers as described in section 2.1.5. Notably, the percentage of FRA purchases relative to total maize production in Zambia has exhibited significant fluctuations on an annual basis, with higher levels observed during election years, such as 2011 and 2015. Furthermore, recent data suggests that FRA purchase prices have been relatively lower than the national average wholesale price, which may have contributed to the decline in FRA purchases. This lack of competitiveness in procurement prices has led to an increasing flow of surplus production through private channels. This observation reinforces the need for our supply chain model to encompass both public and private traders.
2.3. Parameter calibration
2.3.1. Demand parameter estimation
The elasticity of demand for the staple composite (ε) has been estimated in the literature (Roberts and Schlenker 2013), which was used in previous regional maize trade models in Sub-Saharan Africa (Porteous 2019). In line with these studies, we adopt a value of ε = −0.066. Furthermore, We assume Cobb–Douglas preferences (σ = 1) for crop consumption, as commonly employed in equilibrium models (Pohit 1997, Hosoe 2004). Cobb–Douglas preferences assume constant expenditure shares across crops, which are represented by the share parameters (αcm
). This study delves into district-level share parameters, which means that we do not explicitly model individual household behavior. However, we do include household-level data in our study through their aggregated characteristics: (a) composition of rural and urban population by district; and (b) the per capita monthly expenditure on maize and cassava for rural and urban stratum for each district. The expenditure refers to total estimated value of consumption from purchase, self-production, and gift specifically. This expenditure is derived from the 2010 Living Conditions Monitoring Survey, a stratified, nationally representative survey conducted by Zambia's Central Statistical Office (CSO) (Central Statistical Office of Zambia 2010). Leveraging these share parameters and average food prices in each district, we calculate the price and consumption composites. Subsequently, we straightforwardly estimate the demand shifter parameter as .
2.3.2. Storage and transportation cost estimation
The monthly real interest rate (r) is used as a measure of the time value of money. We obtain the r from the World Bank (World Bank 2022), which is estimated by adjusting the lending rate for inflation. For maize, we utilize the storage cost data from Porteous (2019), which is reported as k = 6.919 USD/metric ton. However, since cassava can be harvested year-round once the roots have matured, we assume zero storage costs for cassava.
To estimate the relative trade cost τmn
, we utilize price data for sugar and Zambia's transportation network. We adopt the approach that states,'If a homogeneous commodity can only be produced in one region, the difference in prices (of that commodity) between the origin region and any other consuming region equals the cost of trading between the two regions'. This approach has been previously applied to India (Donaldson 2018) and Peru (Sotelo 2020) due to lack of crop transport data. In Zambia, over 90% of sugar is produced by Zambia Sugar Plc, whose factories operate in Mazabuka, located in the southwest of the country (Kalinda et al
2014). By using trade cost represented by the price difference of sugar between the origin (Mazabuka) and destinations (), we associate the relative trade cost with the effective distance, aiming to estimate the relative trade cost for other market pairs, specified in equation (10)

where effective distance (de ) represents a weighted distance along the 'lowest-effective distance route' between origin and destination, using any available modes of transportation. We estimate the parameters β0 and β1 through ordinary least squares regression. p denotes the wholesale sugar price and the subscripts d and o represent the destination and origin.
The effective distance de can be expressed as:

The weighted ratio vector (,
,
) is determined using nonlinear least squares through grid search. We find the α vector that minimizes the sum of squared residuals for
by recomputing the lowest-effective distance routes for each trail.
To construct the transportation network of Zambia, we utilize the OpenStreetMap database (OpenStreetMap contributors 2022) as detailed in the supplementary information (SI). The weights used to calculate the shortest path are determined by multiplying the weight ratio α by the physical length of the road or railway. Specifically, for railways,
for trunk, primary and secondary roads,
for tertiary and unclassified roads. We fix
as 1 and vary
and
until the minimum root-mean-square error (RMSE) is obtained. The workflow for calculating the relative trade cost between districts within Zambia is summarized in figure 5.
Figure 5. Workflow for calculating the relative transportation cost between districts within Zambia.
Download figure:
Standard image High-resolution imageWe assume that the optimal α vector and corresponding β derived from sugar prices also apply to maize and cassava. To obtain the per unit (additive) trade cost for each crop and trading pair, we multiply the τmn with κc . The trade cost multiplier κc for each crop c is determined through grid searching, aiming to achieve the best fit between the trade model price output and observed prices.
2.4. Counterfactual analysis
To assess the potential impact of transportation infrastructure improvement, we conduct a counterfactual analysis. In this counterfactual scenario, we reduce all market–market trade costs (excluding the international links) to one-half of the initial estimate while maintaining consistency in the remaining parameters and input data with the baseline model.
3. Results
Model results for the four main endogenous variables—crop price (maize only), crop transportation (maize, cassava), crop consumption (maize, cassava), and crop storage (maize only) - are presented in the following sub-sections.
3.1. Price
Modeled maize prices are compared with data from CSO to assess the performance of the model. The mean absolute error (MAE) and RMSE between recorded CSO prices and modeled prices for all districts over time are 0.069 and 0.089 USD kg−1, respectively. According to CSO data, the average maize price is 0.215 USD kg−1. Therefore, on average, the model output's deviation from the surveyed value represents about 33% of the average surveyed value. We also examine the time series of modeled maize price output and survey prices for individual districts. The average correlation within-series is 0.242 (ranging from −0.78 11 to 0.66). Figure 6 presents the goodness of fit results for each district. The model exhibits better performance in the southern districts of Zambia, characterized by higher correlation and lower MAE and RMSE. Overall, our model satisfactorily represented the surveyed price data and effectively captured the maize supply chain in Zambia.
Figure 6. Correlation, mean absolute error (MAE), and root mean square error (RMSE) between modeled and surveyed maize price by district.
Download figure:
Standard image High-resolution image3.2. Trade flows
3.2.1. Trade costs
Trade costs are crucial parameters calibrated in this study. The grid search yields an α vector of , indicating that transportation via the main road system incurs higher costs compared to the railway. Additionally, transport through informal roads, which typically connect smaller towns and villages, involves significantly higher costs than through the main roads that link larger towns. The unit trade cost in Zambia ranges from 0.032 to 0.72 USD km−1t−1. The average trade cost for maize was 0.092 ± 0.062 USD km−1t−1. According to the USDA's 2016 Grain Truck and Ocean Rate Advisory Report (USDA 2016), the national average long-haul grain transportation cost for the United States in 2015 was 0.033 USD km−1t−1. It is not surprising to see higher trade costs in Zambia compared to the United States, considering the less developed transportation infrastructure in Zambia compared to the United States. Moreover, a high degree of spatial heterogeneity in unit trade costs is observed, resulting from uneven infrastructure development. The railway serves as a vital north–south artery through central Zambia, complemented by a dense distribution of main roads that surround it. As a result, these areas experience relatively lower transport costs.
3.2.2. Domestic regional trade
Figure 7 presents that both private and public flow of maize in Zambia concentrates near urban districts, including prominent cities such as Lusaka, Kitwe, Ndola, Kabwe. The trade is also flourishing around the Nakonde region situated near international ports. The FRA plays a crucial role in the management of food distribution by actively purchasing surplus food from various districts within the Eastern Province. These surplus food supplies are then efficiently transported to the central hub in Lusaka, where they are further distributed to meet the demands of the population. It is worth noting that the major food flows observed in Zambia often coincide with the presence of the country's extensive railway network. The railway network serves as a critical artery, allowing for the swift and cost-effective transportation of agricultural products from surplus-producing districts to the central hub and beyond. The annual trade flow results are presented in supporting information (SI)
Figure 7. Comparison of two models of domestic maize flows within Zambia. The top row illustrates model results from this study; the bottom row shows estimates of Porteous (2019). The lighter lines in the top row flow maps represent the private trade flows, while the darker lines represent the public trade flows. The flow direction is from the blue point to the orange point.
Download figure:
Standard image High-resolution imageBecause there is no empirical information on intra-regional trade in Zambia to validate our model results against, we compare our regional trade flow output with another modeled regional flow developed by Porteous (2019) for Sub-Saharan Africa. We aggregate our district-level flow data to ensure consistency with the coarse spatial scale of Porteous (2019)'s 12 . The comparison is displayed in figure 8. Note that Porteous (2019) presents results for 2003 to 2012, so we show the overlapping years in figure 8.
Figure 8. Comparison of two models of domestic maize flows within Zambia. The top row illustrates model results from this study; the bottom row shows estimates of Porteous (2019).
Download figure:
Standard image High-resolution imageThe two model results are of the same order of magnitude (the width of the lines in figure 8 represents the mass of flow). Both (Porteous 2019) and our results observes maize transport from southwestern Zambia to Lusaka market shed and from Solwezi market shed to Kitwe market shed. Maize flows from in-surplus areas to places where demand is higher or actively served as international export hubs. Another important flow observes in our model result is the two-way flow between Mongu market shed and Lusaka/Solwezi market shed. This is mainly due to the different maturity times of maize in different regions in our model. The Mongu region, despite its low production, produced some irrigated maize around January, and this costly maize flows to the Lusaka and Solwezi regions where consumption is high. Most of the maize in other regions matures between May and July, and the cheap maize flow back to the Mongu region.
3.2.3. International trade
In our analysis, we compare the flow results of international trade (trade between Zambia's districts and the aggregated 'Rest of World' node) with the BACI dataset, which is an international trade database for the product-level. The BACI datasets utilize raw data from UN COMTRADE database and address inconsistent declarations of exporters and importers (Gaulier and Zignago 2010). Figure 9 presents a comparison of Zambia's international import and export of maize between the BACI datasets and our model's output.
Figure 9. Comparison of the international export and import between BACI and our estimates.
Download figure:
Standard image High-resolution imageBoth the BACI datasets and our model results confirm that Zambia is a net maize exporter, as the volume of international exports surpasses the international imports. The model's export results are generally higher than the BACI export for most years. This disparity can be attributed to the fact that the BACI dataset only tracks formal trade between countries, whereas African countries have a long history of unreported informal trade. In certain years, our model estimates exports to be considerably higher than the BACI records, even up to 15 times greater volumes. These instances of low formal BACI exports often coincide with periods of government-imposed export bans, which are not explicitly restricted in the model. However, export bans in Zambia exist even though there is a surplus of domestic production. Taking the year 2013 as an example, despite normal production levels and the FRA releasing a large quantity of stocks that year, the government maintained an export ban. The absence of a viable domestic market might compel private traders to export food to other countries leading to the high international export amount as shown in our model, indirectly supported by past news reports of grain traders requesting the government to reverse the ban on exporting maize (Lusakatimes 2013). Furthermore, our price results closely approximate survey prices, implying the persistence of informal and/or illegal exports during export ban periods to maintain price levels.
3.3. Storage
Figure 10(A) depicts the FRA maize storage through time. The amount of maize purchased during the harvest season and released during the lean season varied from year to year, ranging from 45 276 tons to 1.45 million tons. Similarly, the total amount of FRA handled stock (open balance + purchases) exhibited year-to-year variations. In 2012, the total FRA handled stock reached its peak at 2.5 million tons of maize, nearly equivalent to one year of Zambia's maize production. The substantial storage in 2012 resulted in significant destruction, as well as large releases of maize in the subsequent two years.
Figure 10. Food Reserve Agency (FRA) government maize storage over time. Open balance, purchase, and destruction amounts are collected from FRA. Release amounts are calculated in our model according to mass balance. Destruction is the total destruction of the preceding year, for example, the year 2013 destruction actually occurs in 2012. (A) National private storage over time. Private storage increases during the harvest season and then declines to almost zero in the lean season (B).
Download figure:
Standard image High-resolution imageIn our model, we incorporate private storage. Figure 10 shows the trend of private storage over time, aggregated across all districts. Private storage shows an increase during the harvest season, followed by a decline to nearly zero during the lean season. Private storage increased as the FRA releases its inventory until the next harvest season. As the FRA releases its inventory, private storage gradually increases until the next harvest season. Notably, the model does not observe any inter-annual private storage.
There are two main reasons captured by the model why private traders may exhibit reluctance to store maize for long periods of time. One is their anticipation of FRA's maize releases during the lean season, which tends to dampen prices and discourage private-sector storage. Additionally, the high cost associated with storage contributes to this pattern.
3.4. Consumption
Consumption patterns in Zambia exhibits seasonal fluctuations. As expected, a higher maize consumption is observed following the maize harvest compared to the lean season (figure 11). This finding aligns with prior research indicating that Zambians often reduce the frequency and quantity of maize meals during seasons of maize scarcity. Cassava has been suggested as a possible substitute for maize, particularly as a crop that can counteract the decline in maize yields under drought (Dorosh et al 2009). Model results confirm an increase in cassava consumption following maize production shortfalls.
Figure 11. National average maize and cassava consumption by month.
Download figure:
Standard image High-resolution imageOver time, maize consumption exhibits a consistent upward trend, whereas cassava consumption remains relatively stable. This pattern can be partially attributed to population growth, which predominantly occurs in the maize-belt regions where dietary preferences favor maize consumption.
We present the average consumption and average reduction ratio of maize and cassava consumption in figure 12. The reduction ratio is defined as the minimum monthly consumption over the maximum monthly consumption within a year for each district. The southern maize-belt tends to consume a similar amount of maize in the lean season as in the harvest season, while the northern region leverages an increase in cassava consumption to reduce maize intake.
Figure 12. Average monthly maize consumption (A), average monthly cassava consumption (B), the average reduction ratio of maize (C), and average reduction ratio of cassava (D).
Download figure:
Standard image High-resolution image3.5. Counterfactual experiment: lower transportation costs
Table 3 summarizes aggregated key metrics changes across all markets and time periods under this lower trade cost counterfactual case.
Table 3. Key indicator changes aggregated across markets and time.
Metrics | Quantity | Percentage(%) |
---|---|---|
Average maize price | +0.014 USD kg−1 | +6.7 |
Average cassava price | +0.002 USD kg−1 | +2.9 |
Maize expenditure | +154.91 million USD | +3.7 |
Cassava expenditure | +29.20 million USD | +8.6 |
Maize revenues | +405.74 million USD | +10.4 |
Cassava revenues | +24.91 million USD | +8.5 |
Transportation cost | −287.10 million USD | −41.5 |
Storage cost | −22.93 million USD | −4.8 |
Domestic inter-market trade | +1.73 million tonnes | +5.5 |
Net maize exports | +0.17 million tonnes | +1.5 |
Indirect utility | +308.36 million USD | +0.1 |
a Aggregate net maize exports refer to the international export minus international imports.
Lower trade costs result in a 6.7 percent and 2.9 percent increase in maize and cassava average prices, respectively. Lower trade costs stimulate Zambia's maize inter-regional trade and international exports, resulting in domestic inter-market trade increasing 5.5 percent and net exports increasing 1.3 percent. The slightly higher average price of maize increased expenditure on grains by 3.7 percent on the one hand, and agricultural revenues from agriculture by 10.4 percent on the other hand. On average, lowering the trade cost in Zambia was more beneficial to producers at the expense of some consumers, increasing consumers' potential expenditure on agricultural products. The indirect utility of Zambia is slightly increased.
We also take a look at the consumption for each district after the 2018/2019 severe drought to further understand the local impact of lower trade costs. Most of southern and western Zambia experienced their lowest seasonal rainfall since the 1980s. The average increase in consumption for these districts is 1.3 percent for the counterfactual case. The transportation infrastructure improvement could not overcome the severe loss during the drought.
In summary, as a production-surplus country, the decrease in transportation cost could increase the national agricultural revenue and utility through promoting intra- and inter-national trade for a normal year. However, the improvement of infrastructure could not directly mitigate the shock of the severe national-wide disaster. To combat the growing climate risk, transportation infrastructure improvements must be combined with production protection strategies such as irrigation agriculture, drought-tolerant varieties and crops, and fertilizer.
4. Discussion
4.1. Model applications
Our model possesses several unique features that make it well-suited for assessing policy and management decisions related to food security in Zambia. Firstly, its high resolution on both spatial and temporal scales allows us to capture the effects of seasonal climate shocks and production inequities on food trade and supply chains across different regions of Zambia. By analyzing the model outputs over time, we can identify the evolution of these shocks and inequities and their impact on the food system at various times of the year.
Secondly, the model incorporates trade costs directly linked to infrastructure. By accounting for the influence of transportation networks, storage facilities, and other infrastructure on trade costs, the model provides insights into how infrastructure development can enhance the efficiency and effectiveness of food trade and supply chains in Zambia. Section 3.2.1 shows the trade cost associated with the transportation infrastructure. There is huge potential for the improvement of the current transportation system. The regional flow estimated by our model could help with the planning of infrastructure development. For example, a large amount of maize is delivered from the southwest to the vicinity of Lusaka, but the roads surrounding this flow consist mainly of secondary roads. Improving these specific links or constructing primary roads or highways nearby could lower the total trade cost.
Thirdly, our model includes both private and public trade channels. This is a critical feature as both channels play important roles in the Zambian food system. By separately modeling both private and public supply chains, our model can provide insights into the strengths and weaknesses of each channel and identify areas where interventions may be needed to improve food security and reduce poverty.
Finally, the model can be extended to other regions with similar data. While specifically developed for the Zambian food system, many other regions in Sub-Saharan Africa region face similar challenges related to food security. The model's focus on infrastructure and trade costs, as well as its inclusion of both private and public supply chains, make it relevant to a wide range of contexts. This is particularly important for policymakers and stakeholders who aim to use the model to inform interventions aimed at improving food security and reducing poverty in their own contexts. By leveraging existing data and modeling tools, our model has the potential to serve as a valuable resource for understanding and improving food systems in various SSA countries.
4.2. Study limitations
There are several limitations in this study. Firstly, we define the administrative division of the district as markets in the model, which may not fully represent the real-world market catchments. In reality, trading preferences may be influenced by proximity or lower trade costs rather than administrative district boundaries. Thus, the spatial boundaries of the administrative district may not align perfectly with the market catchment. However, adopting administrative divisions as market catchments aligns with the division method employed by the FRA. Treating districts as market catchments also facilitates communication between researchers and the decision-makers, as it corresponds to data collection and administration practices.
Secondly, consumption behaviors are not adjusted over time in this study due to limited district-level consumption data. Instead, we assume that the elasticity of substitution, share parameters, and demand coefficients remain constant throughout the study period. This assumption is supported by the relatively stable annual maize and cassava production (see figure 3) and consumption ratios (CSO 2020). Considering that consumption preferences tend to be sticky with time, our assumption of a CES between the crops is justified and aligns with existing research (Dorosh et al 2007, Porteous 2019).
Thirdly, the construction of the model could be improved by considering the following two crucial aspects of reality in the future. (1) Incorporating more food commodities : only the consumption of maize and cassava is considered in this study. These staple foods account for approximately 70 percent of calorie intake for Zambians and provide a solid basis for capturing food security in Zambia. However, it is also important to consider how farmers use the income from sales to diversify their food consumption from staples meals. Incorporating more food commodities into our supply chain model should be considered in the future when more consumption, production and trade data on other food commodities become available. (2) Incorporating production as an endogenous variable: In this study, we treat crop production as an external variable, because our research question focuses on the transportation of staple crops, rather than farmer production decision. However, research that aims to understand the interact of production decision-making with the full supply chains, could build upon our modeling framework by endogenizing production in future studies.
Fourthly, we encounter data-related limitations. (1) Despite utilizing data from reputable organizations such as the Zambia CSO and the World Bank, there remains a concern regarding the reliability of the data due to potential errors in sampling, measurement, and human entry processes. (2) There is a lack of validation data specifically for the domestic agricultural product supply chain in Zambia. The lack of surveyed regional trade information is one of the reasons for this model. Consequently, our validation process involved comparing model output prices with surveyed price data, the model international trade data with the self-reported international data, and the district-level domestic supply chain with the low-resolution model result. While the sparsity of price data in some districts and unreliable self-report international trade data might make the validation in question, these data are already the most widely used.
These limitations should be considered when interpreting the findings and implications of our study. Future research should strive to address these limitations to further enhance the accuracy and applicability of modeling efforts in the field of food security.
5. Conclusions
This study presents the development of a high-resolution agricultural supply chain model for Zambia. The model effectively simulates the district-level monthly consumption, trade, and storage dynamics of maize and cassava in Zambia. The public maize procurement is distributed to different markets through a routing scheme, while regional maize and cassava trade flows through private channels are estimated through a partial equilibrium model. Analyzing the high-resolution outputs reveals that food consumption is seasonal but varies based on local consumption preferences. Maize consumption peaks during the maize harvest season, while cassava serves as a substitute staple and is predominantly consumed during the lean season. The model output indicates a lack of inter-annual storage of maize in the private channel, likely due to cost constraints and competition from government stocks.
The study also highlights the relatively high trade costs in Zambia. Maize has a mean unit trade cost of 0.092 USD km−1t−1 compared to 0.033 USD km−1t−1 in the United States, a country known for its robust transportation infrastructure. High transaction costs impede regional food flows as most crop transportation only takes place between the Central, Copperbelt, and Lusaka provinces, which are relatively well-connected by transport infrastructure. A counterfactual analysis with lower transportation costs reveals that reducing trade costs increases total net agriculture revenue and total maize and cassava expenditure. Consequently, farmers would benefit more than consumers from the lower trade costs associated with improved infrastructure. These results shed light on the importance of transportation infrastructure in enhancing efficiency in supply chains and reducing costs within the agricultural sector.
Food security remains a critical concern in Sub-Saharan Africa. Food supply chains play a vital role in meeting food security objectives alongside productivity improvements in agriculture. The high spatial and temporal resolution model developed in this study provides a framework for quantifying the direct effect of infrastructure improvements on food availability and costs. Future research can further explore opportunities to improve food supply chain outcomes to inform decision-making.
Acknowledgments
This material is based upon work supported by the National Science Foundation Grant No. CBET-1844773 ('CAREER: A National Strategy for a Resilient Food Supply Chain'), DEB-1924309 ('CNH2-L: Feedbacks between Urban Food Security and Rural Agricultural Systems'), and CBET-2115405 ('SRS RN: Multiscale RECIPES (Resilient, Equitable, and Circular Innovations with Partnership and Education Synergies) for Sustainable Food Systems'). Any opinions, findings, and conclusions or recommendations expressed in this material are those of the author(s) and do not necessarily reflect the views of the National Science Foundation. The authors are grateful for the constructive feedback of anonymous reviewers that strengthened this paper.
Data availability statement
The data cannot be made publicly available upon publication because they are owned by a third party and the terms of use prevent public distribution. The data that support the findings of this study are available upon reasonable request from the authors.
Footnotes
- 6
Private traders are assumed to be price takers under the perfect competition model.
- 7
This 'perp' notation (
) indicates a complementarity condition, which requires that both equation hold and at least one follows equality.
- 8
FRA does not necessarily own the warehousing space; it may be leased. Irrespective of the precise storage ownership structure, the maize stocks are under public control and managed according to the official price policy.
- 9
The initial unit for maize is Zambian Kwacha per meda. A meda is a common measure of weight in Zambia and corresponds to about 17.4 kg of maize. The unit for cassava in the raw price data is Kwacha per kg.
- 10
In this model, local maize prices are treated as endogenous variables, while the international grain price is an exogenous variable. The different setting is because the production of Zambia has little influence on global grain prices.
- 11
Despite unsatisfactory results for Lufwanyama, the CSO provided only 6/132 maize price points for Lufwanyama district over 11 years. This missing data restricts our ability to determine model performance in this district.
- 12
Coarser market catchments in Porteous (2019) are named as XX market shed to distinguish them from districts.
Supplementary data (2.7 MB PDF)